Do Different Value-Added Models Tell Us the Same Things?
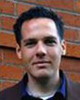
Dan Goldhaber
Director
Center for Education
Data & Research
Professor
University of
Washington-Bothell
Dan Goldhaber
and Roddy Theobald
Highlights
- Statistical models that evaluate teachers based on growth in student achievement differ in how they account for student backgrounds, school, and classroom resources. They also differ by whether they compare teachers across a district (or state) or just within schools.
- Statistical models that do not account for student background factors produce estimates of teacher quality that are highly correlated with estimates from value-added models that do control for student backgrounds, as long as each includes a measure of prior student achievement.
- Even when correlations between models are high, different models will categorize many teachers differently.
- Teachers of advantaged students benefit from models that do not control for student background factors, while teachers of disadvantaged students benefit from models that do.
- The type of teacher comparisons, whether within or between schools, generally has a larger effect on teacher rankings than statistical adjustments for differences in student backgrounds across classrooms.
Introduction
There are good reasons for re-thinking teacher evaluation. As we know, evaluation systems in most school districts appear to be far from rigorous.[1] A recent study [2] showed that more than 99 percent of teachers in a number of districts were rated “satisfactory,”[3] which does not comport with empirical evidence that teachers differ substantially from each other in terms of their effectiveness.[4],[5] Likewise, the ratings do not reflect the assessment of the teacher workforce by administrators, other teachers, or students.[6]
Evaluation systems that fail to recognize the true differences that we know exist among teachers greatly hamper the ability of school leaders and policymakers to make informed decisions about such matters as which teachers to hire, what teachers to help, which teachers to promote, and which teachers to dismiss. Thus it is encouraging that policymakers are developing more rigorous evaluation systems, many of which are partly based on student test scores.
Yet while the idea of using student test scores for teacher evaluations may be conceptually appealing, there is no universally accepted methodology for translating student growth into a measure of teacher performance. In this brief, we review what is known about how measures that use student growth align with one another, and what that agreement or disagreement might mean for policy.
What Do We Know About This Issue?
There is a growing body of research that compares estimates of teacher quality produced by different models. These models, which consider student growth on standardized tests, fall roughly into four categories: “value-added models” that do not control for student background; models that do control for student background; models that compare teachers within rather than across schools; and student growth percentile (SGP) models, which measure the achievement of individual students compared to other students with similar test score histories.[7]
Multiple modeling options for estimating teacher quality
School districts and states that want to use student test scores to inform teacher evaluations have a number of options. There are five large vendors that use varied approaches to translating student growth information into measures of teacher quality (see Table 1).[8] The methods used by three of them—the Value Added Research Center (VARC) at the University of Wisconsin, the American Institutes for Research (AIR), and Mathematica Policy Research—are difficult to summarize because each vendor tailors the approach for each client. But, generally, they all use value-added models.[9]
Table 1: Large Vendors that Estimate Teacher Effectiveness Using Student Test Scores
Vendor | Name of Model | Brief Description |
American Institutes for Research (AIR) | Varied | In most situations, models control for student background |
Mathematica | Varied | In most situations, models control for student background |
National Center for the Improvement of Educational Assessment (NCIEA) | Student Growth Percentile (SGP) Models | Models a descriptive measure of student growth within a teacher’s classroom |
SAS | EVAAS | Models control for prior test scores but not other student background variables |
Value Added Research Center (VARC) | Varied | In most situations, models control for student background |
Value-added models are statistical models that generally try to isolate the contributions to student test scores by individual teachers or schools from factors outside the school’s or teacher’s control. Such factors may include prior test scores, poverty, and race. For instance, students in poorly financed schools, whose parents are not engaged in their education, often do poorly on tests; the value-added model controls for these sorts of factors.[10] There is debate [11] over whether value-added models accurately capture the true contributions (in statistical parlance, “causal estimates”) of schools and teachers as opposed to simply identifying correlational relationships.[12]
The approaches of the other two primary vendors—SAS and the National Center for the Improvement of Educational Assessment (NCIEA)—are easier to define, since each specializes in a specific model. SAS uses the Education Value Added Assessment System (EVAAS).[13] The value-added models discussed in the academic literature tend to include controls for the socio-economic and demographic characteristics of students in order to account for achievement gaps between certain groups. EVAAS, by contrast, intentionally omits these controls. One justification for the omission is that including demographic characteristics differentiates expectations for students in certain groups. (We return to this important distinction below in the secion on What can’t be resolved by empirical evidence on this issue?)
NCIEA takes a completely different approach with the Student Growth Percentile (SGP) model,[14] often called the “Colorado Growth Model.” Both the SGP model and value-added models use a statistical technique called regression to analyze the test score histories of students. Where value-added models purport to separate the contributions of teachers from other variables, the SGP model provides a student growth percentile for each student that shows their growth relative to other student with similar test-score histories. Therefore the SGP model is focused at the student level, and individual student scores are aggregated to the classroom level to obtain a measure of teacher performance; typically the median (or mean) student growth percentile for each teacher’s students becomes the teacher’s SGP score (the “average” growth of the teacher’s students). Outputs from both types of models are currently being used as part of teacher evaluations systems. By design, SGP models do not purport to provide causal estimates of teacher effectiveness (though this does not necessarily imply that they are less accurate measures); they are intended as a descriptive measure of what is – of test score gains relative to other students who scored similarly in the past.”[15]
Different models’ approaches to isolating the effect of the teacher
Given the many options, school districts and states likely have a number of questions about which model is “right” for them. Which model, if any, provides a fair estimate of a teacher’s contribution to student learning? In fact, do value-added estimates provide causal estimates at all? These are heated questions in the field, and they are unlikely to be resolved without more data. But different value-added models make different assumptions about how much variation in test scores should be attributed to teachers. The SAS EVAAS model removes variability due to students’ previous test scores. The three other models also control for previous scores, but they often control for other factors, as well: Mathematica’s DC IMPACT model removes variability due to differences in student background,[16] Mathematica’s Pittsburgh model removes variability due to average classroom characteristics, and AIR’s Florida VAM removes variability that may be due to school-wide factors. Again, NCIEA’s SGP model, instead of removing variability statistically, explicitly controls for student background by comparing only students with similar test score histories. The extent to which these different modeling decisions matter depends on three factors: the correlation between each of these variables and student achievement; how inequitably students are distributed across different classrooms and schools; and how inequitably teacher quality is distributed within and across schools.[17] We return to this discussion in the section on What can’t be resolved by empirical evidence on this issue?
Correlations between value-added estimates from different models
A question that can be answered with empirical data is the extent to which estimates from selected models correlate with each other.[18] Many studies [19] have calculated high correlations (mostly greater than 0.9) between estimates from models that control only for prior student test scores (such as SAS EVAAS),[20] control for student background (such as DC’s IMPACT), and control for average classroom characteristics (such as Pittsburgh’s system). Importantly, two studies [21] calculate higher correlations between estimates that use different models than between estimates that use different exams.
It is only recently that researchers have begun to compare estimates generated by traditional value-added and SGP models. Wright [22] compares SGP estimates to estimates produced by the SAS EVAAS approach, while Goldhaber and colleagues [23] compare SGP estimates to estimates from the full range of value-added models discussed above. Both studies find what might be seen as surprisingly high correlations (around 0.9) between estimates from value-added and SGP models.[24] We say “surprisingly” both because the two types of models have different motivations and because the kinds of student background variables that are in value-added models are often found to influence student achievement.[25]
Most model options result in estimates that correlate highly with one another; however, there is a critical decision that results in estimates with far lower correlations – how teachers should be compared to each other. The two most common choices are to compare teachers across all schools in a district, or to compare teachers only to other teachers in the same school. A few studies [26] compare estimates from these two types of models and find correlations closer to 0.5.
There are two potential explanations for why within-school comparisons change estimates of teacher effectiveness so much. First, schools themselves may make significant contributions to student learning that get attributed to teachers when we don’t account for school factors. Alternatively, teacher quality may be inequitably distributed across schools, meaning that below-average teachers with a lot of below-average peers look a lot better when comparisons are made within schools, while above-average teachers with a lot of above-average peers look worse.[27] Given the trade-off between these two factors, it is difficult to know which type of model is “better.”[28] We will address this question more fully in Section IV.[29]
The impact of model choice on teachers’ effectiveness ratings
While the correlations tell us the degree of agreement of effectiveness estimates, they do not provide the kind of contextual information that individual teachers likely care about. Specifically, teachers want to know how they would rank under different modeling approaches and in what effectiveness category they would fall.[30]
We illustrate the relationship between model correlation and teacher classification in Table 2.[31] In particular, we place teachers into performance quintiles [32] based on how they would rank under different models and compare that rating to the ratings that would result for the same teachers under different models.[33] Panels A-C represent math performance and Panels D-F represent reading performance. Each panel compares the rating of teachers using a value-added model with prior test scores and student covariates to placements from another model: (1) a value-added model that includes only a prior test score (Panels A and D); (2) the SGP model (Panels B and E); and (3) a value-added model that makes within-school comparisons (Panels C and F).[34]
Table 2: Transition Matrices and Correlations for Different Effectiveness Estimates
Panel A. Math | Correlation = 0.97 | VAM with prior test score | ||||
Q1 (Lowest) | Q2 | Q3 | Q4 | Q5 (Highest) | ||
VAM with prior test score and student covariates | Q1 (Lowest) | 17.2% | 2.7% | 0.0% | 0.0% | 0.0% |
Q2 | 2.7% | 13.7% | 3.6% | 0.1% | 0.0% | |
Q3 | 0.1% | 3.4% | 12.9% | 3.5% | 0.0% | |
Q4 | 0.0% | 0.2% | 3.4% | 13.8% | 2.6% | |
Q5 (Highest) | 0.0% | 0.0% | 0.1% | 2.6% | 17.3% | |
Panel B. Math | Correlation = 0.91 | Student Growth Percentiles | ||||
Q1 (Lowest) | Q2 | Q3 | Q4 | Q5 (Highest) | ||
VAM with prior test score and student covariates | Q1 (Lowest) | 15.4% | 4.2% | 0.4% | 0.0% | 0.0% |
Q2 | 4.1% | 10.2% | 5.0% | 0.6% | 0.0% | |
Q3 | 0.6% | 5.0% | 9.4% | 4.7% | 0.4% | |
Q4 | 0.0% | 0.9% | 5.3% | 10.2% | 3.6% | |
Q5 (Highest) | 0.0% | 0.0% | 0.5% | 4.2% | 15.3% | |
Panel C. Math | Correlation = 0.55 | VAM with within-school comparison | ||||
Q1 (Lowest) | Q2 | Q3 | Q4 | Q5 (Highest) | ||
VAM with prior test score and student covariates | Q1 (Lowest) | 9.0% | 5.6% | 3.1% | 1.6% | 0.8% |
Q2 | 5.0% | 5.4% | 4.6% | 3.3% | 1.7% | |
Q3 | 3.1% | 4.4% | 5.0% | 4.5% | 3.0% | |
Q4 | 1.9% | 3.0% | 4.5% | 5.5% | 5.0% | |
Q5 (Highest) | 0.9% | 1.5% | 2.7% | 5.2% | 9.4% | |
Panel D. Reading | Correlation = 0.91 | VAM with prior test score | ||||
Q1 (Lowest) | Q2 | Q3 | Q4 | Q5 (Highest) | ||
VAM with prior test score and student covariates | Q1 (Lowest) | 15.4% | 4.0% | 0.5% | 0.1% | 0.0% |
Q2 | 4.0% | 10.4% | 4.7% | 0.9% | 0.1% | |
Q3 | 0.5% | 4.7% | 9.6% | 4.6% | 0.6% | |
Q4 | 0.1% | 0.8% | 4.7% | 10.5% | 3.9% | |
Q5 (Highest) | 0.0% | 0.1% | 0.5% | 4.0% | 15.4% | |
Panel E. Reading | Correlation = 0.81 | Student Growth Percentiles | ||||
Q1 (Lowest) | Q2 | Q3 | Q4 | Q5 (Highest) | ||
VAM with prior test score and student covariates | Q1 (Lowest) | 13.8% | 4.5% | 1.4% | 0.2% | 0.0% |
Q2 | 5.5% | 7.3% | 5.3% | 1.7% | 0.2% | |
Q3 | 1.8% | 5.0% | 7.2% | 4.6% | 1.4% | |
Q4 | 0.4% | 2.0% | 5.7% | 7.1% | 4.8% | |
Q5 (Highest) | 0.0% | 0.4% | 1.8% | 4.8% | 12.9% | |
Panel F. Reading | Correlation = 0.52 | VAM with within-school comparison | ||||
Q1 (Lowest) | Q2 | Q3 | Q4 | Q5 (Highest) | ||
VAM with prior test score and student covariates | Q1 (Lowest) | 8.8% | 5.5% | 3.0% | 1.7% | 1.2% |
Q2 | 4.9% | 5.5% | 4.6% | 3.1% | 2.0% | |
Q3 | 3.2% | 4.4% | 4.9% | 4.4% | 3.2% | |
Q4 | 2.0% | 3.0% | 4.5% | 5.4% | 4.8% | |
Q5 (Highest) | 1.2% | 1.6% | 3.0% | 5.4% | 8.8% |
If there were complete agreement between two different approaches to estimating teacher performance, we would expect each of the shaded boxes along the diagonal to contain 20 percent of the teachers, since both models would perfectly agree on how to rate each teacher. Clearly this is not the case for any of the comparisons. And, importantly, even models that are very strongly correlated—such as math value-added models with and without student covariates in (Panel B, r = 0.97)—show considerable movement between quintiles. For example, of the teachers identified in the bottom quintile by the value-added model with student covariates, over 13 percent move out of the bottom quintile when we control for student covariates. The movement becomes more pronounced as the correlations decrease, both as we compare the value-added model with student covariates to SGP models and within-school models, and as we make the same comparisons in reading. Strikingly, about 6 percent of teachers who are placed in the top quintile in reading by the value-added model with student covariates are placed in the bottom quintile by the value-added model that makes within-school comparisons, and vice versa.
The differential impact of model choice on teachers’ effectiveness
Correlations also do not distinguish among the types of teachers affected by different types of models. Of particular concern is whether one model or another unduly affects teachers who work primarily with disadvantaged students. Table 3 [35] compares the average percentile rankings of teachers in the most advantaged classrooms to the average percentile rankings of teachers in the least advantaged classrooms for different estimates of teacher effectiveness.
Table 3 demonstrates that SGP and value-added models that do not control for student covariates systematically favor teachers in advantaged classrooms. In reading, for example, the average percentile ranking for teachers in advantaged classrooms is 58.2 compared to 43.6 for teachers in disadvantaged classrooms when teacher effectiveness is calculated with a value-added model that does control for student covariates. But the gap is markedly wider for SGP models and value-added models that do not control for student covariates beyond previous test scores; it is 66.6 compared to 33.8 for SGP estimates, and 71.8 compared to 29.0 for value-added estimates that control only for prior student achievement.[36]
Table 3: Average Percentile Rankings in Advantaged and Disadvantaged Classrooms
Panel 1: Math | Advantaged | Disadvantaged |
Student Growth Percentiles | 60.7 | 41.1 |
VAM with prior test score | 65.1 | 38.2 |
VAM with prior test score and student covariates | 57.8 | 47.7 |
VAM with prior test score, student, and classroom covariates | 60.1 | 46.6 |
VAM with within-school comparison | 51.9 | 48.7 |
Panel 2: Reading | Advantaged | Disadvantaged |
Student Growth Percentiles | 66.6 | 33.8 |
VAM with prior test score | 71.8 | 29.0 |
VAM with prior test score and student covariates | 58.2 | 43.6 |
VAM with prior test score, student, and classroom covariates | 60.3 | 42.8 |
VAM with within-school comparison | 51.0 | 49.4 |
What More Needs to be Known on This Issue?
We have discussed what is already known about how estimates from these models agree with each other and which teachers are affected by any differences that exist. There is an emerging consensus over the answer to the first question; the answer to the second is more preliminary. But in both cases, findings will have to be validated across different states and contexts.
There is another question that could be answered with the right empirical data: what evaluation systems that use student test scores actually lead to greater changes in student performance? Districts and states are presumably adopting new evaluation policies because they believe these policies could lead to better student achievement. Different places have adopted markedly different models, each with its own consequences and rewards. While much of the discussion has focused on teacher bonuses and dismissals, many districts are considering other uses of value-added models, including tying evaluation scores to professional development.[37] Once a few years have passed, researchers will be able to determine whether any of these systems have led to changes in the teacher workforce or in student achievement.
What Can’t be Resolved by Empirical Evidence on This Issue?
There are three important questions about the use of student growth measures that cannot easily be answered with existing empirical evidence:
- Which model produces estimates that are the “fairest” to individual teachers?
- What is the appropriate balance between accuracy and transparency for evaluation systems that use student test data?
- Is it more appropriate to compare teachers within schools or across schools?
But, the three comparisons in Table 2 provide case studies for each of these questions.
The question of “fairness” is likely to be at the heart of any debate about teacher evaluation, and the debate over controlling for student characteristics other than prior test scores shows why it is hard to know which model is more “fair” to individual teachers. As we have seen, the evidence demonstrates that teachers who teach in advantaged classrooms benefit from models that do not control for student covariates like race and poverty, but policymakers may be reluctant to employ models that do control for these factors. This is because, given what we know about the relationship between these variables and student achievement, the model would expect low-income students to show lesser gains than high-income students. So a teacher of disadvantaged students could get a higher value-added measure than a teacher of advantaged students even though her class showed less actual growth. On the other hand, this sort of outcome may seem perfectly fair given that some teachers face far greater obstacles than others given the readiness to learn of the students in their classrooms. Moreover, many administrators are understandably loath to use an evaluation system that may discourage teachers from working in disadvantaged classrooms.[38] It is purely a judgment call about which result is fairer to individual teachers and how it may affect achievement goals. What is fair to teachers may not be fair to students, and vice versa.[39]
Differences between value-added and SGP rankings (Panels B and E of Table 2) illustrate the potential tradeoff between transparency and accuracy in an evaluation system. SGPs may not be designed to give causal estimates of teacher effectiveness, but they are understandably appealing to many policy makers and administrators because of the transparency of the resulting scores. It is much easier to explain to parents, for example, that a teacher’s score is the median estimate of student growth in her class as opposed to a coefficient from a linear regression.[40] Again, we can argue that value-added estimates may be better designed to give causal estimates of teacher quality, but whether the transparency of SGPs outweighs this benefit is another matter for policy makers and administrators to judge.
Finally, the issue of whether to compare teachers within or across schools (see Panels C and F of Table 2) is another situation for which empirical evidence provides little guidance. With just a single year of data [41] there is no way to know whether differences in student performance across schools are due to school factors or differences in the average quality of teachers. A model that compares teachers to the average teacher across all schools produces estimates of teacher effectiveness that are combinations of teacher and school effects on student achievement. But a model that compares teachers to the average teacher within a school assumes that teacher quality is distributed evenly across schools. It may also lead to competition rather than cooperation between teachers. Given that statistical models simply cannot distinguish between teacher and school effects with just one year of data, policymakers and administrators again must decide which model is most appropriate.
Practical Implications
How Does This Issue Impact District Decision Making?
Our reading of the research shows that modeling choices do have an important impact on teacher rankings.[42] Of models that control for prior achievement, the high correlations between those that do and do not explicitly account for student characteristics might suggest that debates over covariate adjustments are misplaced. But high correlations can, as we show above, mask pretty large differences in the rankings of teachers in different classrooms. We would argue that the differences are meaningful enough for policymakers to be concerned when deciding what model to adopt.
The evidence about the impact of model specification on performance rankings raises another issue for consideration. Most districts and states have only just begun to use student growth measures to inform high-stakes decisions about teachers. It is likely that their methods for doing so will change. New York State, for example, plans to report SGP scores to teachers in 2011-12 and value-added scores beginning in 2012-13 in certain subjects and grades.[43] The empirical evidence suggests that, depending on the model adopted, this change could have consequences for individual teachers. So even though eachof these evaluation systems may be superior to those now used elsewhere, the potential shifts in teacher rankings could serve to undermine the usefulness of both.[44]
The data needed to estimate teacher performance based on student growth are now widely available. This means that administrators who use these measures for high-stakes purposes could be confronted by teachers who could rightly argue, and point to empirical evidence, that their ranking would have been different under different assumptions. One cannot escape the fact that different models lead to different results, but the issue of how they do could be made clear to everyone when the models are being adopted. Transparency would encourage buy-in at the start and help prevent surprises later.[45]