How Stable are Value-Added Estimates across Years, Subjects, and Student Groups?
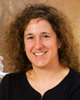
Susanna Loeb
Professor of
Education
Stanford University
Faculty Director
Center for Education
Policy Analysis
Co-Director
PACE
Susanna Loeb
and Christopher A. Candelaria
Highlights:
- A teacher’s value-added score in one year is partially but not fully predictive of her performance in the next.
- Value-added is unstable because true teacher performance varies and because value-added measures are subject to error.
- Two years of data does a meaningfully better job at predicting value added than does just one. A teacher’s value added in one subject is only partially predictive of her value added in another, and a teacher’s value-added for one group of students is only partially predictive of her valued-added for others.
- The variation of a teacher’s value added across time, subject, and student population depends in part on the model with which it is measured and the source of the data that is used.
- Year-to-year instability suggests caution when using value-added measures to make decisions for which there are no mechanisms for re-evaluation and no other sources of information.
Introduction
Value-added models measure teacher performance by the test score gains of their students, adjusted for a variety factors such as the performance of students when they enter the class. The measures are based on desired student outcomes such as math and reading scores, but they have a number of potential drawbacks. One of them is the inconsistency in estimates for the same teacher when value added is measured in a different year, or for different subjects, or for different groups of students.
Some of the differences in value added from year to year result from true differences in a teacher’s performance. Differences can also arise from classroom peer effects; the students themselves contribute to the quality of classroom life, and this contribution changes from year to year. Other differences come from the tests on which the value-added measures are based; because test scores are not perfectly accurate measures of student knowledge, it follows that they are not perfectly accurate gauges of teacher performance.
In this brief, we describe how value-added measures for individual teachers vary across time, subject, and student populations. We discuss how additional research could help educators use these measures more effectively, and we pose new questions, the answers to which depend not on empirical investigation but on human judgment. Finally, we consider how the current body of knowledge, and the gaps in that knowledge, can guide decisions about how to use value-added measures in evaluations of teacher effectiveness.
What is the Current State of Knowledge on This Issue?
Stability across time
Is a teacher with a high value-added estimate in one year likely to have a high estimate the next? If some teachers are better than others at helping students improve their performance on standardized tests, we would expect the answer to be “yes.” But we also know that teachers are better at their jobs in some years than in others, so we would not expect their value added from one year to the next to be exactly the same. That is, part of a teacher’s influence on students persists over time, and part of it varies. It may vary for a range of reasons. Professional development might lead to improvement, or family responsibilities or school reforms might cause distractions in one year but not in another.
Instability is not necessarily a bad thing; if a school is engaging in substantial change, one might expect teacher performance to change as well. In addition, value-added measures are subject to error because the students have good or bad days when taking the test. The smaller the number of test scores used to create value-added measures, the more important students’ idiosyncratic performance is likely to be. So, we can think of value-added estimates as measuring three components: (1) true teaching effectiveness that persists across years; (2) true effectiveness that varies from year to year; and (3) measurement error. The relationship between a teacher’s value added in one year and her value added in another year combines all three of these factors.
One statistic that describes the year-to-year persistence in value-added estimates is the correlation coefficient, a measure of the linear relationship between two variables. The correlation coefficient is 1.0 if a teacher’s value-added measure in one year is perfectly predictive of her score in the following year and zero if her score one year tells us nothing about how she will fare the next. A study of elementary school teachers found correlations from one year to the next of 0.6 to 0.8 for math and 0.5 to 0.7 for reading.[1] Other studies have found lower correlations, ranging from 0.2 to 0.6, depending on location, school level and the specifications of the value-added model.[2] These estimates are similar to the year-to-year performance correlations found for other occupations, including college professors, athletes, and workers in insurance sales.[3] Overall, a teacher’s value-added estimate in one year provides information about his or her value added in the following year, but it is not perfectly predictive.
While one year of data does only a modest job of predicting teachers’ future value added, averaging the value-added measures across more than one year makes predictions more accurate. Using the same data and model specification, one study found a correlation of 0.4 comparing one year of data to the next and a correlation of 0.6 using two years of data.[4] In other words, in this study, two years of data does a meaningfully better job at predicting value added than does just one. It is not as clear from the literature whether more than two years of data is helpful. The one available study found that the correlation of two years of value-added data to future value-added data was significantly higher than one year, but that additional years of data (up to six) added only a little more predictive power.[5]
Thought of in another way, teachers who have a high value-added estimate in one year are likely to have a high value-added estimate in the next, but some teachers with high value-added scores in one year have quite low scores in the next. Table 1 presents a transition matrix that displays the value-added ranking of one sample of teachers over two years.[6] Teachers are grouped into quintiles based on their value-added score in each year. Half of teachers in the top fifth in the first year remained there, while 8 percent dropped all the way to the bottom fifth. We also see that 43 percent of teachers who had value-added estimates in the bottom quintile in one year remained there the next. If there were no relationship between years, we would expect only 20 percent of teachers to be in this group since they would be evenly divided between the five quintiles.[7] The table shows some stability in the value-added measures as most teachers either remain in the same quintile from one year to the next or move just one quintile up or down.
Table 1: Stability of Teacher Value-Added Across Time
(Percent of Teachers by Row)
Ranking in year 2 (Quintiles) | |||||
Ranking in year 1 (Quintiles) |
Q1 (Bottom) | Q2 | Q3 | Q4 | Q5 (Top) |
Q1 (Bottom) | 43% | 29% | 14% | 10% | 4% |
Q2 | 26% | 21% | 25% | 18% | 9% |
Q3 | 12% | 21% | 28% | 25% | 15% |
Q4 | 10% | 19% | 19% | 28% | 23% |
Q5 (Top) | 8% | 11% | 11% | 19% | 50% |
Notes: Total teachers = 941.Source: Koedel & Betts (2007) |
The stability of value-added measures differs between teachers. Teachers who improve more will have lower year-to-year stability. Difference in the number of students a teacher teaches each will also affect stability[8] because it affects the reliability of the measure. A recent study finds evidence that teachers who teach different types of students also systematically differ in the stability of their value-added estimates. In particular, the study found that value-added stability is lower when students are low test performers and when a greater proportion are poor (eligible for subsidized lunch), Hispanic or black.[9]
Stability across subjects
Teachers may teach multiple subjects over the course of a year, and they might be better at teaching one subject than another. For example, in elementary school, some teachers might be more effective teaching multiplication than reading. If a teacher who is very good at teaching one topic is also very good at teaching another—if her value-added measures are similar across topics—we might be comfortable using value-added measures based on a subset of student outcomes, for example, just math or just reading. However, we might be less comfortable using a single measure of student outcomes if the value-added estimates varied a lot across topics. If it did, we would want to measure teacher effectiveness in each subject that we care about.
To assess the consistency of teacher effectiveness across subjects, we can compare a teacher’s value added in one subject to her value added in another. The consistency of these measures across subjects, similar to consistency over time, combines both true differences in effectiveness and measurement error. That is, one reason a teacher’s value-added estimates are not perfectly correlated across subjects is that both the estimates are measured with error. Another reason is true differences in contributions to student learning.
Studies that have looked at teachers’ consistency across reading and math have found correlation coefficients in the range of 0.2 to 0.6—similar to the correlations for the year-to-year comparisons.[10] To help make these findings clearer, we created a matrix based on the data from one of these studies.[11] The matrix in Table 2 shows rankings of teachers on both their reading and math value-added scores. We see that 46 percent of teachers who ranked in the bottom fifth in math also ranked in the bottom fifth for reading. Similarly, 46 percent of teachers who ranked in the top fifth for math also ranked in the top fifth for reading (compared with only four percent in the bottom fifth). The matrix suggests that, on average, teachers who are effective in math are also effective in reading, although some teachers are better at one than the other.
Teachers may differ in their value added not only across subject areas but within subject areas. Many standardized exams are composed of tests within tests. For example, the math section of the Stanford Achievement Test contains one subtest on procedures and one on problem-solving. Two studies have examined the consistency of value-added estimates based on these subtests, and one found correlations within the range of 0.5 to 0.7, while the other found correlations within the range of 0.0 to 0.4.[12] We have seen that the range in correlations across years and between subjects is due largely to differences in the methods for estimating value added. But in the second study, which looks within subjects, the lower correlations come from differences in data sources and differences across the subtests of the Stanford Achievement Test.[13]
Table 2: Stability of Teacher Value-Added Across Subjects
(Percent of Teachers by Row)
Ranking in reading (Quintiles) | ||||||
Ranking in math (Quintiles) |
Q1 (Bottom) | Q2 | Q3 | Q4 | Q5 (Top) | Total |
Q1 (Bottom) | 46.4% | 27.3% | 14.1% | 8.7% | 3.6% | 704 |
Q2 | 23.2% | 28.0% | 22.3% | 17.4% | 9.2% | 622 |
Q3 | 16.4% | 24.5% | 22.8% | 20.9% | 15.5% | 580 |
Q4 | 8.3% | 17.5% | 24.7% | 28.1% | 21.4% | 612 |
Q5 (Top) | 4.1% | 9.5% | 16.1% | 24.8% | 45.5% | 589 |
Total | 641 | 671 | 616 | 608 | 571 | 3,107 |
Source: Loeb, Kalogrides, & Béteille (forthcoming) |
Stability across student populations
The final type of consistency that we address is that which crosses student populations. Despite the great variation among student groups – demographic, academic, and otherwise – few studies have examined the extent to which teachers are more effective with one group of students than with another. Understanding these differences helps tell us whether a teacher who is effective with one group is likely to be effective with another. It also tells us how important it is to calculate value-added measures by student sub-group.
Two studies have compared teachers’ value added with high-achieving students to the same teachers’ value added with lower-achieving students. Both studies find that teachers who are better with one group are, in most cases, also better with the other group. The first study calculates two estimates: one based on the test scores of the teachers’ high-performing students and the other based on the scores of their low-performing students.[14] While the samples used in the analyses are small, the authors find a correlation between the estimates of 0.4. As with the cases discussed above, the differences could come from variations in teachers’ true value-added across student groups or from measurement error enhanced by the small sample size. The second study uses a different approach to ask a similar question. It finds that approximately 10 percent of the variance in teachers’ value-added estimates can be attributed to the interaction between teachers and individual students, indicating substantial similarity in teacher effects between groups.[15]
Another study conducted a similar comparison of teachers’ value added with English language learners and English-proficient students.[16] The authors found a correlation between teachers’ value-added measures with the two groups of 0.4 to 0.6 for math and 0.3 to 0.4 for reading. For comparison, and to distinguish measurement error from true differences in teacher effectiveness, the authors ran similar correlations with randomly separated groups of students. They found correlations of 0.7 for math and 0.6 for reading — evidence that teachers’ value added for English learners is similar to their value added for students who already speak English, though again, they found that some teachers are somewhat better with one group than with the other. Table 3 provides a matrix similar to those described above. It shows that 50 percent of teachers in the bottom fifth in value added for math with English-proficient students are also in the bottom fifth with English learners. That compares to less than four percent of teachers who are in the top fifth with English learners.[17] Similarly, 59 percent of the teachers in the top fifth in value added for math with English-proficient students are also in the top fifth in valued added with English learners; less than four percent are in the bottom fifth. Thus, the relationship between teachers’ value-added measures for these student groups is at least as strong as the relationship between value-added measures across years and subject areas.
Table 3: Stability of Teacher Value-Added Between English Learners and Others (Math)
Ranking for Non-English Learners (Quintiles) | ||||||
Ranking for English Learners (Quintiles) |
Q1 (Bottom) | Q2 | Q3 | Q4 | Q5 (Top) | Total |
Q1 (Bottom) | 49.90% | 25.10% | 14.80% | 7.10% | 3.70% | 467 |
Q2 | 22.70% | 32.40% | 22.90% | 14.10% | 5.40% | 480 |
Q3 | 15.10% | 23.10% | 27.50% | 20.60% | 11.80% | 484 |
Q4 | 8.60% | 15.10% | 24.60% | 30.20% | 19.90% | 480 |
Q5 (Top) | 3.70% | 4.40% | 10.20% | 28.00% | 59.20% | 475 |
Total | 409 | 525 | 541 | 504 | 407 | 2,386 |
Source: Loeb, Soland, and Fox (2012) |
What More Needs to be Known on This Issue?
We have summarized the research on how well value-added measures hold up across years, subject areas, and student populations, but the evidence is based on a relatively small number of studies. Future research could better separate measurement error from true differences; more systematically compare estimates across model specifications; identify clear dimensions of time, topic, and student populations; and provide evidence on the sources of instability.
Again, we see changes in teachers’ value-added estimates partly because the measurements are imprecise. While numerous papers have highlighted this imprecision, most studies of instability have not systematically considered the role of measurement error in estimates aside from the type that is caused by sampling error. Measurement error can come from many sources including errors in the initial tests; the fact that many value-added estimates are based on small samples of students; errors in the data systems such as incorrect student teacher matches; effects of differential summer learning; and multiple support teachers working with same students.
We also recommend further research on the differences that exist when value added is measured across datasets and model specifications. This is described by Dan Goldhaber’s brief in this series.[18] With further study, researchers could clarify the role played by the model specifications and datasets in instability, and they could compare stability across a set of specifications. Then they could repeat the analysis across a range of datasets.
Our understanding of value-added consistency could also benefit from the examination of more subject areas and more student groups. We compared math to reading, but we could also consider outcomes in writing, science, social studies, and other topics. Across student groups, we could consider achievement levels and behavioral tendencies, as well as background characteristics such as gender, race/ethnicity, and family income.
Finally, further research could help uncover the causes of the inconsistency in value-added measures. To what degree are year-to-year changes due to differences in teacher effectiveness that schools can actually control? To what extent do factors outside the classroom affect teacher performance? To what extent are teachers handicapped simply because they aren’t aware of the resources available to help students with different needs? When schools understand the causes of inconsistency in value-added measures, they not only learn about the appropriate use of these measures, they also learn how they can best help their teachers improve.
What can’t be resolved by empirical evidence on this issue?
It is clear from the research so far that value-added measures will never be completely stable across time, topics, or student groups, nor would we necessarily want them to be because true teacher effectiveness likely varies across these dimensions. Given these general findings, there are a number of questions that do not depend on empirical evidence. They include choices about the number of years of data to combine, the subject areas or topics to measure, and the student groups among which to distinguish.
As described above, there are substantial yearly differences in teacher value-added scores. Districts can learn more about future value added by looking at more years of initial value added.[19] However, while using additional years leads to more stability, it also restricts the sample of students for whom these measures are available because teachers have to teach for multiple years to have multiple years of data. Deciding what level of instability is acceptable is a decision for practitioners, not researchers.
While instability across subject areas is perhaps not as great as instability across years, it is still substantial. It matters a lot which tests form the basis of the value-added measures. Do they measure math or reading? Do they measure factual knowledge or reasoning skills? Researchers can help develop more reliable tests, but the choice of subject areas and the tradeoffs across types of measures are matters of judgment. Similarly, considering groups of students separately increases instability, but if there are groups in which a district has a particular interest, it might be worth the cost in stability for the benefit of targeting this group.
More generally, while research can evaluate the effectiveness of policies that use value-added measures, research can never determine the optimal approach for a given district or school.
Practical Implications
How Does This Issue Impact District Decision-Making?
Year-to-year instability calls for caution when using value-added measures to make decisions for which there are no mechanisms for re-evaluation and no other sources of information. Similarly, the gain in stability from using multiple years of data points to benefits of using multiple years of data (at least two). However, even with multiple years of data, the instability can be consequential, a problem that points to supplementing value-added measures with other information.
The instability across subjects also has important implications. First, it matters what is on the test. It should cover the skills and knowledge that are valued. Topic inconsistency can also have implications for teacher assignment and development. Also knowing how teachers perform on different outcome measures, educational leaders can more carefully target professional development.
Finally, the consistency across student groups can affect practical decisions. By knowing how teachers perform on different outcome measures, education leaders can better target professional development to teachers’ needs and can better target teachers to classes or subject areas for which they are effective. If teacher effectiveness varies a lot across subjects, leaders might make more careful distinctions among teaching jobs so teachers are teaching the subjects at which they are best. Moreover, if researchers can identify, in particular, why some teachers are more effective with under-served populations, school reform efforts might greatly benefit.
In summary, value-added measures vary for the same teacher from year to year, subject to subject, and student group to student group. Although the similarity across measures suggests they are useful tools for decision-making, the variation suggests the benefit of being able to update decisions when new information becomes available; if a teacher appears relatively ineffective in one year, it is worth seeing her measure in another year as well. Instability in value-added measures argues for using them in relatively low-stakes decisions that carry opportunities for reconsideration.